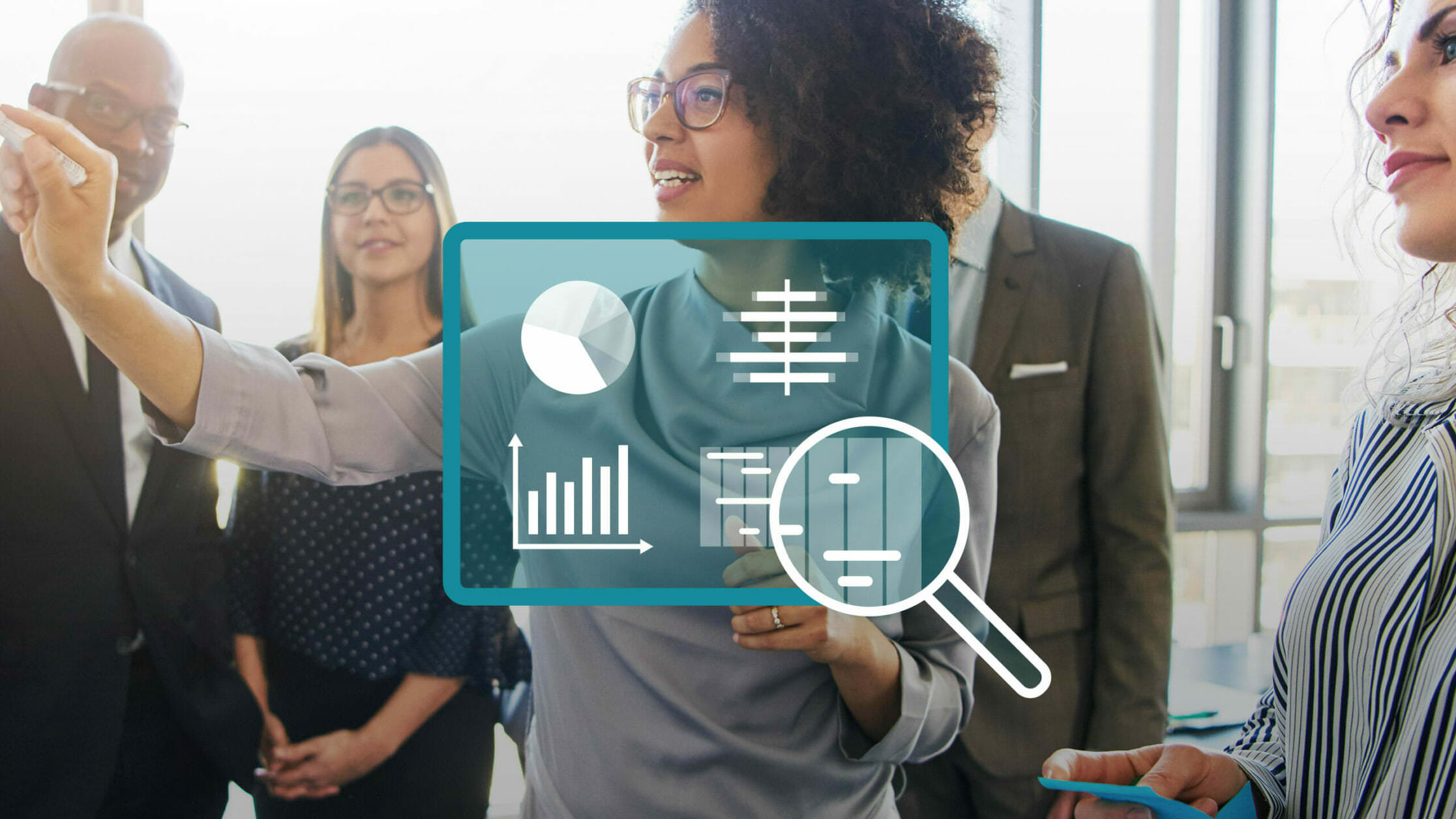
Five Steps in Learning to Speak the Language of Data Visualization Technologies
This content was previously published by Campus Labs, now part of Anthology. Product and/or solution names may have changed.
As a twenty-first-century consumer my home is full of sophisticated technology that is deceptive in its simplicity. On a given day, I may use my smart speakers to get directions, find a recipe, or – in one instance my wallet is unlikely to forget - adjust the thermostat. Those of us in higher ed who use data visualizations technologies may experience a similar tension between sophistication and simplicity.
In both instances, to learn something new or to achieve a desired result requires simply to ask. It is the art of “asking correctly,” wherein the challenge lies. An ask that is made in a way that is outside of how the technologies understand will quickly lead to unexpected complications.
As higher education professionals, we bring human distinctions and nuances with us whenever we interact with data visualization software. In a recent conversation I had with a well-intentioned senior leader about his institution’s data, he said, “I just need to know how well we’re doing. Our students, faculty, staff, curriculum – how good are they?” A fair inquiry, but one asked in a way that makes visualizing data a challenge. Just as my smart speaker cannot know which of the lights in my home I am asking it to turn on without my clarification, a data visualization program needs similar elaboration to run a successful query: How was he defining “well” or “good”? What metrics was he using in pursuit of those definitions? What were his faculty, staff, or students “good” at?
Given our company’s expertise in establishing data cultures at colleges and universities, this was not my first experience engaging with a campus leader who wanted to be able to use data visualizations and dashboards to understand the wellbeing of his institution, but who did not know how to state what it was that he wanted to learn. My colleagues and I have written previously about the data governance framework we recommend establishing to use data for continuous improvement, and the best practice lessons we have learned in our efforts. In both posts, we discussed the need to establish clearly known priorities. Doing this will create the clarity needed in our language and help reduce the risk that we conduct needless analyses or report on whatever happens to seem interesting, without a larger purpose.
Our team has put together a resource to help higher ed professionals clearly state what questions they have of their data so that they can use visualization technology to learn and act. Let’s review the five steps of establishing an analytic priority to visualize:
1. Identify the Problem or Phenomena
In theory, this is the easy part. Most institutions abound with common narratives about what is going well on their campus and what needs improvement. Notice, though, that this is the same positive/negative binary that the senior leader I mentioned earlier struggled with. Let me be clear: it is logical to want to improve positive aspects and minimize negative, but we want this to be a general starting point, which we then filter to be more specific.
For example, the common narrative on a campus may be that “retention needs improvement.” Successful analytics require that we better articulate the problem/phenomena. It is a clearer starting point to state that “enrollment trends at our institution indicate that retention of first year students into their second year has been declining since 2016.”
2. Choose a Focus Area
Remember my earlier comments about “asking” technology correctly: I can ask a smart speaker to play “music” which will work, but without a focus, the algorithm will data-dredge and take random guesses at what I will find interesting. I will have more specific results if I ask for a given genre, artist, album, or song.
Similarly, with higher ed analytics, one can run a query about student retention, but one will quickly be awash in information that proves to be a struggle to make actionable, impactful meaning of. One learns more by focusing on something specific, such as the efficacy of specific student success interventions in correlation to student retention.
3. Identify Items to Analyze
With a problem/phenomena stated and an area of focus identified, one can consider what items from this area generate data to visualize and analyze. It may seem obvious, but is worth stating: it is impossible to visualize and analyze data that does not exist. Arriving at this moment will either be an affirmation of the data collection efforts your campus has invested in or a call to action to pause and to change your practices to ensure you will have the data you need for your focus area moving forward.
Following the retention example, an institution may identify items such as early course registration, targeted engagement outreach, or first year experience course outcomes by focusing on student success interventions.
4. Generate Questions, One Item at a Time
If a campus has been diligent in data collection efforts, it is tempting at this point to throw all the data together and see what meaning comes out. Patience must be exercised, though: an abundance of data does not inherently produce an abundance of meaning.
At this stage, we suggest being methodical in producing a series of analytic questions for each of the items your institution has generated data on related to your focus area. Following our retention example, one may ask a whole series of questions:
- Are first year students who participate in early registration retained at a higher rate than their peers who register later in the term?
- Are first year students who participate in early registration enrolling in high DFW courses at the same frequency as their peers who register later in the term?
- Does participation in early registration show an impact on performance of course learning outcomes?
5. Refine a Question Which You Can Act Upon
Each of the questions above challenges us to view an item from a slightly different angle and consider what actionable steps we might take from what we learn. Further refinement of our language tasks us to go beyond colloquial language (the same challenge my senior leader faced) and state explicitly what we wish to know of our data:
- Unrefined: Are first year students who participate in early registration retained at a higher rate than their peers who register later in the term?
Consider: In this question, how are first year students defined? How is early registration defined? Precision in our definitions allows for greater accuracy in our query.
- Refined: Excluding students who entered the institution with 15+ transfer credits, are first year students who participate in early registration (within the first two weeks of registration for the next term) retained at a higher rate than their peers who register later in the term?
While this question would be a mouthful for one person to ask another, an analytic inquiry will respond with precisely the desired information and that your institution can respond to accordingly.
It can be easy to forget that our engagement with data visualization technology truly does require fluency in speaking the language of data. Our daily consumer technologies generally do a good job of hiding this reality from us – they act as a translator because they have a fiscal incentive in anticipating our asks, inquiries, and interests. When faced with analyzing our own institutional data, no such translator exists, and it is up to us as agents of change at our institution to learn how to “ask correctly.”